In a paper published in Science on February 1, 2024, researchers at New York University report that AI can use the fragmented experiences of a child to discern pieces of information about the world around it, such as learning there is something called a crib, or matching words to images in a book.
Between 6 and 9 months old, kids start picking up their first words and connecting them to what they see. The big question is: How much of this learning comes from just seeing things and using basic learning, and how much needs a more specific kind of learning?
For years, scientists have been trying to understand how children’s minds take shape through carefully controlled experiments involving toys that allow scientists to probe when cognitive skills develop. Studies have shown that 16-month-old babies can use statistical reasoning to determine when toys are broken, and babies as young as 5 months understand object permanence. In addition, some individual babies have been closely followed over time by Deb Roy, a scientist at the Massachusetts Institute of Technology and director of its Center for Constructive Communication, who set up overhead cameras in all the rooms of his house in 2005 and recorded his son’s linguistic development, providing valuable data that detailed the evolution of words. That work suggested it was not how many times a word was repeated that predicted whether Roy’s son learned it early but whether it was uttered in an unusual spot in the house, at a surprising time, or in a distinctive linguistic context.
The innovative use of headcams has given researchers an even more intimate view of early childhood.
In this study, researchers recorded one child from 6 to 25 months old using a head-mounted camera. For a year and a half, a baby named Sam wore a headcam in weekly sessions that captured his surroundings. They then taught a regular neural network computer program, using 61 hours of videos showing how words and visuals matched up. The program learned to connect words to things in the child’s daily life, even handling new things it had not seen before. This shows that important parts of how we learn the meaning of words can happen by connecting what we see and what we hear based on the experiences of just one child’s data.
This information is one part of a much larger pursuit to build an AI that can mimic a baby’s mind. If researchers were able to accomplish this, it would be revolutionary to cognitive science and would help researchers to understand human development. It could also potentially lead AI to help humans teach new skills in a more intuitive way.
But first, back to babies. Babies are the opposite of a chatbot (large language models), learning words not by rapidly digesting all the world’s texts, but by being in the world itself through sensory input and play.
“By our calculations, it would take a child 100,000 years of listening to spoken words to reach the word count” of the training sets for chatbots, said Brenden Lake, a computational cognitive scientist at NYU who led the study. “I was also skeptical that those [chatbot] models would shine a lot of light on human learning and development.”
To gather the data for their study, researchers utilized an innovative approach involving headcams worn by babies. Since 2013, families have contributed to the SAYCam database, recording audiovisual experiences of babies between 6 and 32 months old. Sam, the subject of this study, provided researchers with 600,000 video frames and 37,500 transcribed words, covering a crucial period of cognitive development.
The AI was trained on just 1% of Sam’s waking hours, demonstrating an impressive ability to match basic nouns and images. However, the study does not claim to resolve the ongoing debate among scientists about the foundational cognitive skills required for language acquisition.
Various theories about how humans learn language exist, with some proposing an innate language ability while others emphasize social or inductive reasoning skills. The AI study suggests that basic associative learning, such as linking a word to its corresponding image, can occur without specialized cognitive machinery.
While the AI showed proficiency in associating simple nouns with images, it falls short of replicating a child’s language learning abilities fully. The study has sparked interest in applying these methods to single-child data sources, providing a unique perspective on language development.
As the researchers continue through the data, the study raises questions about the limitations of the current AI model. Can AI progress beyond simple nouns to grasp verbs, prepositions, or social expressions? Despite the strides made, the AI’s ability to learn complex linguistic structures remains an open question. As the quest for a more child-like AI continues, the study serves as a crucial step toward the mysteries of human language.
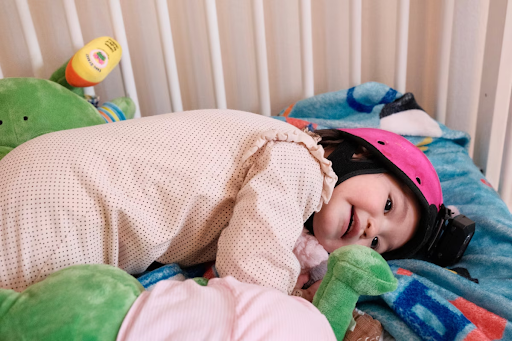
Photo Courtesy of The Washington Post